Jan Seuntjens
PhD, University of Ghent
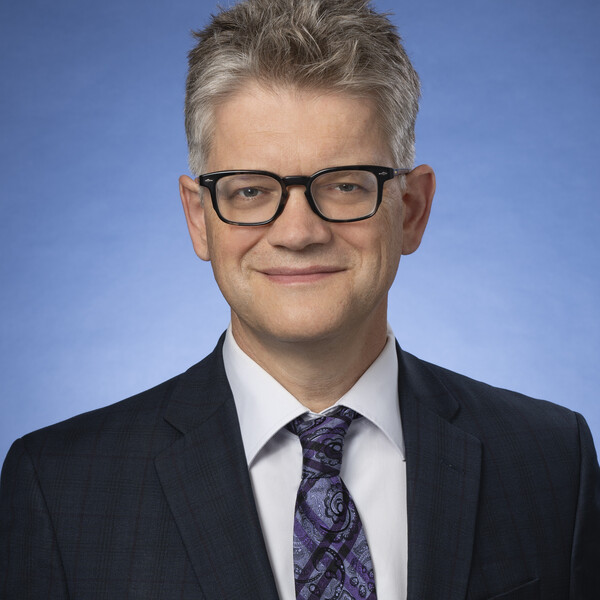
At a Glance
- Development of radiation medicine treatment technologies and modalities including mixed-beam electron-photon radiation therapy and low-cost particle therapy technology development.
- Novel radiation detectors and treatment delivery verification technologies focusing on calorimetry, Cherenkov and nanoparticle-based dosimetry and computational methodologies
- Monte Carlo calculation techniques at the macroscopic and microscopic scale
- Data-driven radiation medicine optimization and the application of AI in optimizing clinical outcome based on data
Short Bio
Dr. Jan Seuntjens (PhD, University of Ghent, Belgium) is a medical radiation physicist with a background in fundamental calorimetric dosimetry and Monte Carlo numerical simulations. He is the Head of Medical Physics at the Orey and Mary Fidani Family Endowed Chair in Radiation Physics at the Princess Margaret Cancer Centre.
Dr. Seuntjens’ research primarily involves techniques to optimize the efficacy of radiation therapy by making the determination and planning of the dose delivered in radiation therapy more accurate. To this end he has developed special Monte Carlo simulation techniques and detection techniques which are now routinely used in clinical practice. A second area of interest is the development of new radiation therapy treatment techniques. These include Modulated Electron Radiation Therapy (MERT), intraoperative radiation therapy techniques (IORT) and, more recently, on technologies that pave the way towards low-cost proton therapy. He has also worked on Monte Carlo computational methods both for clinical dosimetry as well as to better understand the mechanistic effect of radiation on cellular response. Finally, his group has used data science methods based on clinical data sources with the ultimate goal to optimize treatment outcome of cancer patients.
Research Synopsis
Theme 1: Novel radiation medicine treatment technologies:
We are working on two novel technologies. The first technology that we invented and that we have been studying the implementation and clinical utility of is mixed photon-electron radiation therapy (MBRT). The MBRT technology is designed to simultaneously take advantage of the characteristics of both types of radiation, photons and electrons, employing the photon collimation and couch motion capabilities of current robotic linac equipment. Although different approaches have been tested and reported in the literature, our group is one of only three groups in the world that have developed this capability to a point where clinical translation has become feasible and logical. The goal of this research is thus to bring to the clinic a new radiation therapy treatment technique that is superior in sparing normal tissues for specific important treatment sites. This research is supported by the foundation grant from the CIHR. The second technology is proton therapy (PT). PT can deliver a high radiation dose that conforms much better to the tumour and delivers from 1.5 to 3 times less dose to normal tissues surrounding the target, compared to conventional RT. In some situations, exposing healthy tissues can be eliminated. As a result, PT has the potential to improve quality of cancer survival and quality of life and significantly reduce the socio-economic burden of treatment. However, PT as it is offered today is expensive, mostly due to the high capital cost of the equipment and substantial operating costs. We have launched project IMPACT with a six-year goal to determine the design parameters of a compact PT system that has capital and operational cost comparable to conventional radiotherapy (i.e., new acceleration technology that can be brought to clinical operation for about 1/6-1/10 of the current PT cost). Project IMPACT is an international, multidisciplinary project that aims to design with consideration of medical risk and health economic implications at reducing side effects and their long-term impact on the patient and on society.
Theme 2. Novel radiation detectors and treatment delivery verification technologies:
The uncertainty of the radiation dose delivered to the patient is determined by uncertainties resulting from several steps in the treatment devices’ calibration and dose delivery. Clinical application of modern radiation therapy delivery technology, such as TomoTherapy, Cyberknife, magnetic resonance image-guided radiation generators (i.e., MRLinacs), volumetric modulated arc therapy, scanned proton beam radiation therapy, etc deliver radiation using small fields in high-dose fractions at possibly extreme dose rates (i.e., FLASH therapy). In theme 2 we aim to reduce these uncertainties by creating and developing new-generation clinically viable detector technologies, by defining new methodologies and by providing data for international clinical dosimetry guidelines. Two main detector methodologies are pursued, calorimetry and Cherenkov radiation detection in three main research objectives: (1) to develop a miniature `Aerrow’ graphite probe calorimeter to address calibration of clinical small photon beams and proton beams; (2) to address inconsistencies in the reference dosimetry of kV intraoperative radiation beams using the Aerrow; (3) to develop a Cherenkov radiation dosimeter technique suitable for in situ clinical dosimetry and high-dose rate FLASH dosimetry. The impact of this program is to bring overall better consistency, conceptual clarity and improved accuracy to the dosimetry of small and nonstandard beams. Improved accuracy in the long term will lead to better outcomes. Our program builds on a track record of practice-changing dosimetry successes with some of our technologies to become available to end-users for testing and implementation.
Theme 3. Data-driven radiation medicine optimization:
Modern health systems generate and store enormous amounts of digital patient information which have the potential to be leveraged to improve and personalize the delivery of medical care. In recent years, adoption of comprehensive electronic health records (EHR) systems and advances in machine learning (ML) and artificial intelligence (AI) have led to numerous promising and potentially clinically applicable examples of the utility of harnessing this growing pool of digital data. Progress has accelerated in the past few years, as advancements in the fields of deep learning (DL) and neural networks have led to progressively more accurate models able to intake and process extremely large, unfiltered and potentially multimodal datasets to predict clinical events, including inpatient mortality, 30-day readmission, length of stay, and discharge diagnoses. In recent years we have worked on prediction models for patient outcomes using analysis of medical images, radiation dose distributions in conjunction with clinical parameters using DL. My group is founding partner of a consortium named MedOmics that has the goal to provide tools and build systems for dynamic patient data collection and analysis using artificial intelligence techniques, initially in the space of radiation oncology. Theme 3 is to deploy MedOmics tools using data at the PMCC and work towards optimization of radiation dose for treatment planning that incorporates all data pieces available on a patient. This paradigm is called outcomes-based treatment planning and represents the holy grail of radiation medicine optimization.
Recent Publications
J. Seuntjens, E. F. Lartigau, S. Cora, G. X. Ding, S. Goetsch, J. Nuyttens, D. Roberge (2017) ICRU Report No. 91 Prescribing, Recording, and Reporting of Stereotactic Treatments With Small Photon Beams, Journal of the ICRU Volume 14 No 2 (2014), Published July 2017.
Renaud MA†, Serban M, Seuntjens J (2019) Robust mixed electron-photon radiation therapy optimisation, Med Phys. 46(3):1384-1396, 2019 Jan 9, doi: 10.11002/mp.13381.
André Diamant†, Veng Jean Heng, Avishek Chatterjee, Sergio Faria, Houda Bahig, Edith Filion, Robert Doucet, Farzin Khosrow-Khavar, Issam El Naqa and Jan Seuntjens (2020) Comparing local control and distant metastasis in NSCLC patients between CyberKnife and conventional SBRT, Radiotherapy and Oncology 144:201-208, doi.org/10.1016/j.radonc.2020.01.017.
Wang XJ, Miguel B, Seuntjens J., Fernandez-Varea JM (2020) On the relativistic impulse approximation for the calculation of Compton scattering cross sections and photon interaction coefficients used in kV dosimetry, Phys Med Biol 2020 Jun 17; 65(12):125010. doi: 10.1088/1361-6560/ab8108.
Mullins J, Renaud MA, Serban M, Seuntjens J. (2020) Simultaneous Trajectory Generation and Volumetric Modulated Arc Therapy Optimization, Med Phys 2020 Jul;47(7):3078-3090 doi: 10.1002/mp.14155.
Heng VJ, Renaud MA, Zerouali K, Doucet R, Diamant A, Bahig H, DeBlois F, Seuntjens J. (2020) Large-scale dosimetric assessment of Monte Carlo recalculated doses for lung robotic stereotactic body radiation therapy, Phys Med 2020 June 19; 76:7-15. doi: 10.1016/j.ejmp.2020.06.006
Zlateva Y, Muir BR, El Naqa I, Seuntjens JP. (2020) Cherenkov emission-based external radiotherapy dosimetry: I. Formalism and feasibility. Med Phys. 2019 May;46(5):2370-2382. doi: 10.1002/mp.13414. Epub 2019 Apr 29.
Peter G.F. Watson, Marija Popovic, Liheng Liang, Nada Tomic, Slobodan Devic, Jan Seuntjens (2020) Clinical implication of dosimetry formalisms for electronic low-energy photon IORT, Practical Radiation Oncology, 2020 August 10. 11(1):e114-e121. DOI: https://doi.org/10.1016/j.prro.2020.07.005