Main Second Level Navigation
- Biomedical Imaging
- Cancer Diagnosis & Therapy
- Cancer Mechanisms & Models
- Cardiovascular Sciences
- Data Science & Computational Biology
- Deep Learning & Artificial Intelligence
- Image-Guided Therapy & Device Development
- Neuroscience
- Stem Cells & Regenerative Medicine
- Structural Biology
- Research Facilities
- Faculty Directory
Deep Learning & Artificial Intelligence
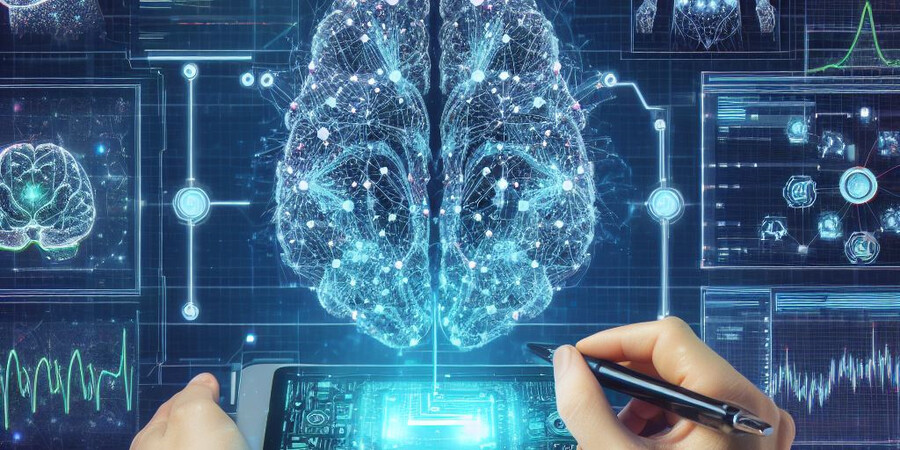
Deep Learning is changing the way we understand diseases, make diagnosis, and treat patients. Armed with vast data sets scientists in the field of Deep Learning and Artificial Intelligence in healthcare leverage techniques rooted in mathematics, statistics, computer science, and machine learning to develop novel algorithms and models that positively impact healthcare. This data encompasses a wide spectrum including medical imaging, omics data, digital pathology, wearables, clinical notes, and more. A significant portion of contemporary research in this domain focuses on developing and applying deep learning algorithms to harness these vast datasets to develop algorithms and models for improving our diagnosis, treatment, and understanding of diseases.
Examples of specific research topics include:
- Disease Diagnosis: Developing AI-driven models to enhance the accuracy and timeliness of disease diagnosis in cancer, cardiovascular, neurological, and psychiatric diseases and more.
- Disease and Outcome Prediction: Developing AI models to predict disease progression, patient risk, and patient outcomes or response to therapy, as well as perform patient stratification for clinical trials.
- Medical Imaging Analysis: Utilizing deep learning for the interpretation of medical images, including radiology, pathology, microscopy, and dermatology, to develop biomarkers, understand disease models, and assist in more precise diagnostics.
- Electronic Health Record (EHR) Mining: Extracting valuable insights with natural language processing from electronic health records to improve patient care, treatment strategies, and healthcare management.
- Telemedicine and Remote Monitoring: Developing AI solutions for remote patient monitoring and telemedicine to enhance accessibility and the quality of care.
- Computational Imaging: Using AI and computational models to facilitate the acquisition and reconstruction of medical images, including modalities such as microscopy, magnetic resonance imaging, ultrasound and computed tomography.
- Ethical and Regulatory Considerations: Investigating the ethical and regulatory challenges associated with the integration of AI in healthcare, including data privacy, bias mitigation, and compliance with healthcare standards.
- Explainable AI (XAI) in Healthcare: Advancing methods to make AI models interpretable and transparent to clinicians and patients to ensure trust and accountability.
View Medical Biophysics faculty working in this area.
View recent research posters from MBP labs working in this area.