Gregory Schwartz
PhD, Drexel University
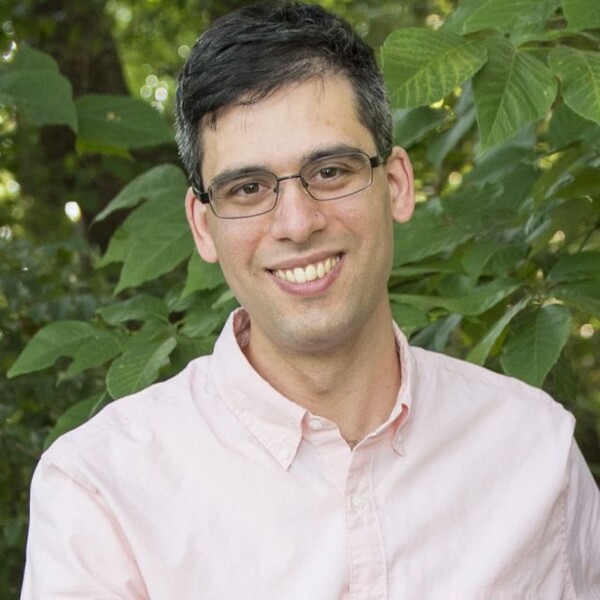
At a Glance
- Our lab has expertise in developing new methods and algorithms for data exploration and visualization
- We utilize and contribute cross-disciplinary techniques from bioinformatics, computational biology, systems biology, machine learning, and more to understand cancer therapeutic resistance.
- We develop methods for multi-omic integration to understand cancer heterogeneity.
- The goal of our research is to develop new analytical approaches and make discoveries that help overcome resistance to cancer treatments.
Short Bio
Dr. Schwartz became interested in heterogeneous systems by studying the diversity and selection of the immune receptor repertoire during his Ph.D research at Drexel University (2011-2016). He subsequently joined the Perelman School of Medicine at the University of Pennsylvania as a postdoctoral researcher, where he studied heterogeneous responses to strong selective pressure in cancer by developing and applying new methods and algorithms to elucidate drug response (2016-2021). He has designed methods including the integration of different data modalities such as transcriptomes and proteomes to discover pan-cancer biomarkers as well as techniques to characterize and quantify new classes of diverse mutations at the nucleotide level in acute myeloid leukemia. More recently, he has leveraged single-cell technologies to deconvolve resistance to targeted therapy in T-cell acute lymphoblastic leukemia.
Research Synopsis
Therapies elicit short-term responses to cancer, but they are commonly followed by the emergence of cells resistant to the treatment. This resistance is facilitated by a diverse population of cells. While we are beginning to understand the extent of heterogeneity in cancer, there are existing knowledge gaps in the strategies to overcome resistance to therapy. We are leveraging new technologies such as single-cell -omics to deconvolve this complex system by outputting large volumes of data per individual cell. However, new methods and algorithms are required to interpret this data. As such, our research program focuses on developing new analytical tools to understand heterogeneous responses to therapy.
In order to accomplish this goal, we are currently focusing on designing algorithms to:
- Integrate multi-omic data to better understand heterogeneity at different data modalities such as the transcriptome, epigenome, and proteome
- Infer cell-cell communication networks between and within cancer cells and the surrounding microenvironment
- Understand clonal shifts in response to therapies over time
- Better visualize complex, multi-omic data in data science
- Help improve patient treatment
Recent Publications
Kahn BM, Lucas A, Alur RG, Wengyn MD, Schwartz GW, Li J, Sun K, Maurer HC, Olive KP, Faryabi RB, Stanger BZ. The vascular landscape of human cancer. J Clin Invest. 2021 Jan 19;131(2). doi: 10.1172/JCI136655. PubMed PMID: 33258803; PubMed Central PMCID: PMC7810485.
Rome KS, Stein SJ, Kurachi M, Petrovic J, Schwartz GW, Mack EA, Uljon S, Wu WW, DeHart AG, McClory SE, Xu L, Gimotty PA, Blacklow SC, Faryabi RB, Wherry EJ, Jordan MS, Pear WS. Trib1 regulates T cell differentiation during chronic infection by restraining the effector program. J Exp Med. 2020 May 4;217(5). doi: 10.1084/jem.20190888. PubMed PMID: 32150623; PubMed Central PMCID: PMC7201917.
Schwartz GW, Zhou Y, Petrovic J, Fasolino M, Xu L, Shaffer SM, Pear WS, Vahedi G, Faryabi RB. TooManyCells identifies and visualizes relationships of single-cell clades. Nat Methods. 2020 Apr;17(4):405-413. doi: 10.1038/s41592-020-0748-5. Epub 2020 Mar 2. PubMed PMID: 32123397; PubMed Central PMCID: PMC7439807.
Schwartz GW, Shauli T, Linial M, Hershberg U. Serine substitutions are linked to codon usage and differ for variable and conserved protein regions. Sci Rep. 2019 Nov 21;9(1):17238. doi: 10.1038/s41598-019-53452-3. PubMed PMID: 31754132; PubMed Central PMCID: PMC6872785.
Petrovic J, Zhou Y, Fasolino M, Goldman N, Schwartz GW, Mumbach MR, Nguyen SC, Rome KS, Sela Y, Zapataro Z, Blacklow SC, Kruhlak MJ, Shi J, Aster JC, Joyce EF, Little SC, Vahedi G, Pear WS, Faryabi RB. Oncogenic Notch Promotes Long-Range Regulatory Interactions within Hyperconnected 3D Cliques. Mol Cell. 2019 Mar 21;73(6):1174-1190.e12. doi: 10.1016/j.molcel.2019.01.006. Epub 2019 Feb 7. PubMed PMID: 30745086; PubMed Central PMCID: PMC6485942.
Schwartz GW, Manning B, Zhou Y, Velu P, Bigdeli A, Astles R, Lehman AW, Morrissette JJD, Perl AE, Li M, Carroll M, Faryabi RB. Classes of ITD Predict Outcomes in AML Patients Treated with FLT3 Inhibitors. Clin Cancer Res. 2019 Jan 15;25(2):573-583. doi: 10.1158/1078-0432.CCR-18-0655. Epub 2018 Sep 4. PubMed PMID: 30181385; PubMed Central PMCID: PMC6335170.
Schwartz GW, Petrovic J, Zhou Y, Faryabi RB. Differential Integration of Transcriptome and Proteome Identifies Pan-Cancer Prognostic Biomarkers. Front Genet. 2018;9:205. doi: 10.3389/fgene.2018.00205. eCollection 2018. PubMed PMID: 29971090; PubMed Central PMCID: PMC6018483.