Sushant Kumar
PhD, Pennsylvania State University
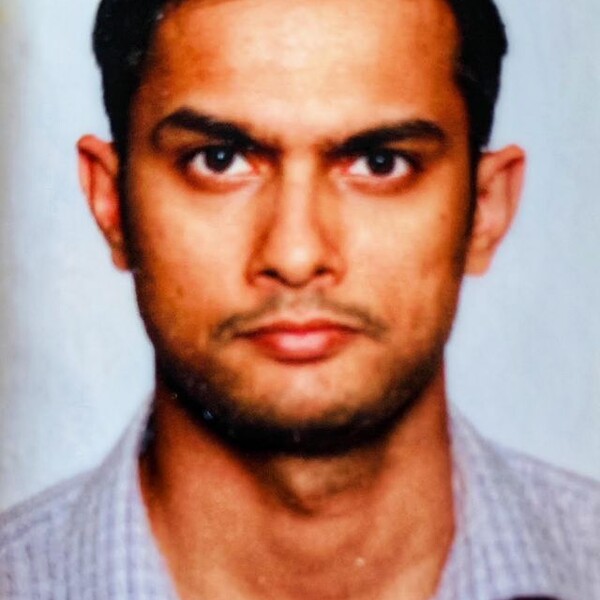
At a Glance
- Integrative computational approaches to study cancer biology on genetic and molecular level
- Development of machine learning models to detect clinically actionable biomarkers in cancer
- Influence of population-specific germline variants on cancer diagnosis, treatment, and drug side effects
- Cooperative interactions on the genetic and cellular level in tumor evolution & metastasis
Short Bio
Dr. Kumar is interested in developing computational methods and tools to obtain molecular- and genetic-level insight into cancer biology. His lab utilizes genomics, machine learning, and biophysics-based approaches to address these questions. Dr. Kumar completed his Ph.D. in bioinformatics and genomics at the Pennsylvania State University. During his Ph.D., he applied biomolecular simulations and structural bioinformatics approaches to study protein folding and binding processes in disordered proteins. As a postdoctoral associate at Yale, he worked extensively on developing integrative approaches to interpret and prioritize genomic variants associated with various diseases, including cancer. Over the years, Dr. Kumar has extensively collaborated with multiple scientists and clinicians at Yale and as part of large-scale genomic consortia (PCAWG, 1000 Genomes, ENCODE, HGSVC, and GSP projects).
Research Synopsis
Rapid declines in sequencing costs have enabled large-scale genome and exome sequencing for various cancer cohorts. A critical shared objective among such studies has been to understand how genomic variants affect tumor etiology. How may we develop robust quantitative models to predict the impact of somatic mutations on gene expression and protein function? Furthermore, how may we leverage these quantitative models to prioritize genomic variants and utilize this knowledge to develop new cancer therapeutics? My lab is interested in developing integrative methods that use multiple data resources and cross-disciplinary approaches to address questions of this nature.
In particular, my lab's current research directions are in the following areas:
Molecular Modeling Methods to Interpret Coding Mutations in Cancer
Exome sequencing remains the primary sequencing platform in cancer studies, owing mainly to its relatively lower costs and strong ability to identify clinically actionable variants. Among coding variants, quantifying the molecular impact and prioritization of missense mutations in cancer cohorts remains challenging. Prior efforts to quantify the impact of missense mutations relied on statistical frameworks that evaluate the positive selection signal for somatic mutations. However, these approaches fail to provide mechanistic insights into how high impact missense mutations drive tumor progression. In our previous work, we have developed methods that integrate protein structure and protein motion information to evaluate molecular impact of cancer mutations and identify cancer driver genes. As a continuation of these works, my lab will develop new integrative machine learning methods integrating protein structure and cancer mutations to identify novel drug targets and predict efficacy & side effects of drugs among cancer patients.
Characterizing Role of Non-coding Mutations and Genomic Rearrangements in Cancer
The overwhelming majority of cancer mutations fall within non-coding regions of the genome. Despite their higher frequency, clear insights into how non-coding mutations play causal roles in various cancer types remain limited. Similarly, current genomic studies primarily focus on understanding the effects of point mutations and INDELs on phenotype while overlooking those of genomic structural variations (SVs), despite the fact that SVs are more likely to affect gene expression and play critical roles in various cancer types. As with non-coding mutations, our understanding of how SVs influence cancer progression remains limited. One of my lab’s primary research goals is to build methodologies for assessing the molecular impact of variants, as well as prioritizing non-coding mutations and SVs in different cancer types.
Integrative Approaches to Study Molecular Etiology of Cancer
Precision medicine initiatives in oncology, such as the cancer moonshot aim to bring better diagnosis and treatment efficacy. Currently, genomics and transcriptomics-based technologies constitute the core of precision medicine in cancer. The majority of these efforts involve generating comprehensive catalogs of driver alterations. Such catalogs have translated into a few instances in which targeting actionable genes leads to therapeutic developments in cancer. However, genomics or transcriptomics alone cannot untangle the intricate relationships between tumor biology and patient outcomes. Previous studies have shown the role of other factors that influence tumor biology, including epigenetics, microbiome, protein modifications, and metabolic factors. Thus, employing integrative omics-based studies constitute a more effective means of understanding tumor biology and guide therapeutic developments in cancer. Toward this goal, my group will develop data-driven methods that utilize multi-omics data to decipher aberrant signaling modules, accurately detect cancer subtypes, and identify new biomarkers underlying tumor growth.
Delineating the Allelic Heterogeneity and Pharmacogenomic Efficacy in Diverse Populations
Over the last decade, large-scale population-level sequencing has led to rapid advances in genomic medicine. However, despite tremendous progress, most genomic studies remain inherently biased toward the European ancestry group. The lack of ethnic diversity in these studies not only poses ethical/moral dilemmas – it also significantly hinders our understanding of the genetic architecture of human disease and the realization of personalized medicine. In particular, allelic heterogeneity leads to differences in the causative mutations in a given gene, which may thus confound cancer's prognosis within a diverse population. To address these challenges, my group is developing methods and tools to comprehensively characterize allelic heterogeneity and elucidate the roles of population-specific variants in various cancer cohorts.
Recent Publications
- Whole-genome sequencing of phenotypically distinct inflammatory breast cancers reveals similar genomic alterations to the more commonplace non-inflammatory breast cancers
Xiaotong Li; Sushant Kumar; Arif Harmanci; …. Naoto T. Ueno; Savitri Krishnamurthy; Lajos Pusztai; Mark Gerstein. Genome Medicine (2021)
doi: https://doi.org/10.1186/s13073-021-00879-x - Haplotype-resolved diverse human genomes and integrated analysis of structural variation
Peter Ebert, Peter Audano, Qihui Zhu, Bernardo Rodriguez-Martin, …. Sushant Kumar ... Tobias Marschall, and Evan E. Eichler. Science (2021)
doi: https://doi.org/ 10.1126/science.abf7117 - SVFX: a machine learning framework to quantify the pathogenicity of structural variants.
Sushant Kumar*, Arif Harmanci*, Jagath Vytheeswaran and Mark Gerstein. Genome Biol 21, 274 (2020).
doi: https://doi.org/10.1186/s13059-020-02178-x (*equal contribution). - Passenger Mutations in More Than 2,500 Cancer Genomes: Overall Molecular Functional Impact and Consequences.
Sushant Kumar*, Warrell J*, Li S, McGillivray P, Meyerson W, Salichos L… Mark Gerstein. Cell (2020).
doi: https://doi.org/10.1016/j.cell.2020.01.032 (*equal contribution). - Discovery and characterization of coding and non-coding driver mutations in more than 2,500 whole cancer genomes.
Esther Rheinbay, Morten Nielsen, Federico Abascal, Grace Tiao, Henrick Hornshøj, Julian M. Hess, Randi Pedersen, Lars Feuerbach, …Sushant Kumar, … Gad Getz, PCAWG Drivers and Functional Interpretation Group, and ICGC/TCGA Pan-Cancer Analysis of Whole Genomes Consortium. Nature (2020).
doi: 10.1038/s41586-020-1965-x - Leveraging protein dynamics to identify cancer mutational hotspots in 3D-structures.
Sushant Kumar, Declan Clarke and Mark Gerstein. Proc. Natl. Acad. Sci. 201901156 (2019).
doi:10.1073/PNAS.1901156116