Benjamin Haibe-Kains
PhD
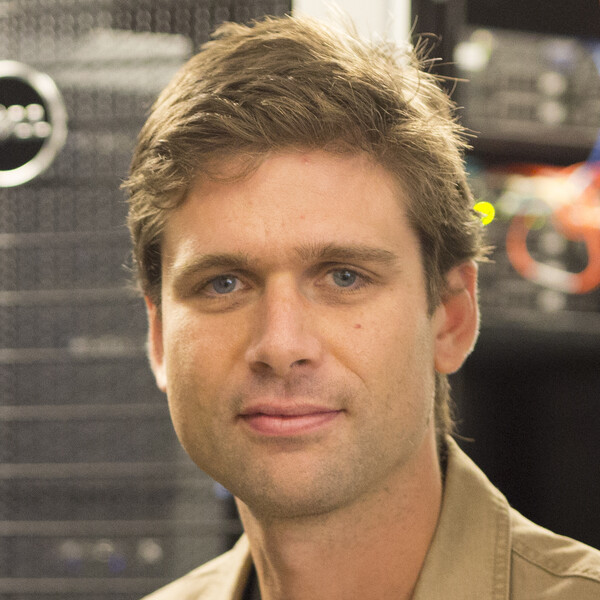
At A Glance
- Focus of the lab includes Computational Biology, Bioinformatics, Machine learning, Cancer Genomics and Pharmacogenomics
- Strong emphasis on translational research, with close collaboration with clinicians to develop relevant biomarkers and novel therapeutic strategies
- Large network of collaborators in multiple cancer types
- Integrative analysis of multi -omics data in different model systems (cell lines, xenografts, primary tumors)
- Algorithmic and software development at the core of the lab research
- We make our research fully reproducible!
Short Bio
Dr. Benjamin Haibe-Kains is a Senior Scientist at the Princess Margaret Cancer Centre (PM), University Health Network, and Associate Professor in the Medical Biophysics department of the University of Toronto. Dr. Haibe-Kains earned his PhD in Bioinformatics at the Université Libre de Bruxelles (Belgium). Supported by a Fulbright Award, he did his postdoctoral fellowship at the Dana-Farber Cancer Institute and Harvard School of Public Health (USA). Dr. Haibe-Kains’ research focuses on the integration of high-throughput data from various sources to simultaneously analyze multiple facets of carcinogenesis. Dr. Haibe-Kains’ team is analyzing large-scale radiological and (pharmaco)genomic datasets to develop new prognostic and predictive models to improve cancer care.
Research Synopsis
Predictors of drug response based on pharmacogenomic data. My laboratory is focusing on developing robust genomic predictors of drug response from pharmacogenomic data. The Cancer Genome Project (CGP) and the Cancer Cell Line Encycolpedia (CCLE) studies recently published drug sensitivity data on large panel of genomically-characterized cancer cell lines with the aim of unraveling new associations between genomic features of these cell lines and their response to drugs. We used CGP data to train genomic predictors of response to 15 drugs screened in both studies, and showed that half of these models could not be validated on CCLE (J Am Med Inform Assoc 2013). We then investigated the consistency between these two large pharmacogenomic studies as potential cause of failure for most of our predictors and discovered that, although gene expression data were highly concordant, the drugs sensitivity data were highly inconsistent across studies (Nature 2013; Cancer Res 2014). We are now developing new analysis pipelines to increase robustness of drug phenotypic measurements to build more robust genomic predictors of drug response.
Large-scale causal gene regulatory networks. With the recent interest in network medicine I decided to investigate the use of gene interactions extracted from the biomedical literature and structured biological databases (referred to as “priors”) to better infer gene-gene interaction networks from gene expression data. I implemented predictionet, a network inference approach integrating genomic data and priors, and developed a new validation framework based on high-throughput perturbation experiments (Genomics 2014). Collaborating with Entagen, I have implemented a web application that enable users to easily infer predictive gene interaction networks by combining interactions extracted from publications, pathway databases and gene expression data (Nucleic Acids Res 2012).
Gene expression-based classification models to robustly identify cancer molecular subtypes. Molecular subtyping of cancers is key in the path of personalized medicine. However the initial cancer subtyping models (I) were not robust when applied to independent datasets; and (II) were based on the expression of large numbers of genes, which complicated their translation into clinical assays. These shortcomings prompted me to develop a simple, yet robust, three-gene classification model for breast cancer molecular subtyping. I showed that this model was more robust than published models while yielding similar prognostic value than more complex gene signatures (J Natl Cancer Inst 2012); I am now working with Caprion Inc. to translate this model into a clinical assay. I have applied a similar approach in ovarian cancer and identified a new angiogenic subtype with significant prognostic value (PLoS ONE 2012). I have developed a novel trans-species classifier of meduloblastoma molecular subtypes (Genomics 2015).
Prognostic biological processes in breast and ovarian cancer subtypes. As numerous microarray datasets are publicly available, I quickly recognize the need for a bioinformatics framework that enables joint analysis of gene expression data generated from different platforms and laboratories. I designed a meta-analysis framework to robustly quantify activity of hallmarks in breast cancer (estrogen and her2 signaling pathways, proliferation, angiogenesis, apoptosis, immune response, and tumor invasion), and assessed their prognostic value in each molecular subtype separately. This framework opened the way for many other studies investigating subtype-specific biomedical questions (Clin Cancer Res 2008). Two of the signatures we have discovered have been patented. I have recently extended my meta-analysis framework to ovarian cancer (PLoS Comput Biol 2013).
Visit publication information for Dr. Haibe-Kains on Google Scholar
Recent Publications
- Evaluation of statistical approaches for association testing in noisy drug screening data. Smirnov P, Smith I, Safikhani Z, Ba-Alawi W, Khodakarami F, Lin E, Yu Y, Martin S, Ortmann J, Aittokallio T, Hafner M, Haibe-Kains B. BMC Bioinformatics. 2022 May 18
- Bimodal gene expression in cancer patients provides interpretable biomarkers for drug sensitivity. Ba-Alawi W, Kadambat Nair S, Li B, Mammoliti A, Smirnov P, Mer AS, Penn LZ, Haibe-Kains B. Cancer Res. 2022 May 10
- PharmacoDB 2.0: improving scalability and transparency of in vitro pharmacogenomics analysis. Feizi N, Nair SK, Smirnov P, Beri G, Eeles C, Esfahani PN, Nakano M, Tkachuk D, Mammoliti A, Gorobets E, Mer AS, Lin E, Yu Y, Martin S, Hafner M, Haibe-Kains B. Nucleic Acids Res. 2021 Nov 26
- Assessing therapy response in patient-derived xenografts. Ortmann J, Rampášek L, Tai E, Mer AS, Shi R, Stewart EL, Mascaux C, Fares A, Pham NA, Beri G, Eeles C, Tkachuk D, Ho C, Sakashita S, Weiss J, Jiang X, Liu G, Cescon DW, O'Brien CA, Guo S, Tsao MS, Haibe-Kains B, Goldenberg A. Sci Transl Med. 2021 Nov 17
- Orchestrating and sharing large multimodal data for transparent and reproducible research. Mammoliti A, Smirnov P, Nakano M, Safikhani Z, Eeles C, Seo H, Nair SK, Mer AS, Smith I, Ho C, Beri G, Kusko R; Massive Analysis Quality Control (MAQC) Society Board of Directors, Lin E, Yu Y, Martin S, Hafner M, Haibe-Kains B. Nat Commun. 2021 Oct 4
- Drug sensitivity prediction from cell line-based pharmacogenomics data: guidelines for developing machine learning models. Sharifi-Noghabi H, Jahangiri-Tazehkand S, Smirnov P, Hon C, Mammoliti A, Nair SK, Mer AS, Ester M, Haibe-Kains B. Brief Bioinform. 2021 Aug 11
- Prediction of Human Papillomavirus (HPV) Association of Oropharyngeal Cancer (OPC) Using Radiomics: The Impact of the Variation of CT Scanner. Reiazi R, Arrowsmith C, Welch M, Abbas-Aghababazadeh F, Eeles C, Tadic T, Hope AJ, Bratman SV, Haibe-Kains B. Cancers (Basel). 2021 May 8
- The impact of the variation of imaging parameters on the robustness of Computed Tomography radiomic features: A review. Reiazi R, Abbas E, Famiyeh P, Rezaie A, Kwan JYY, Patel T, Bratman SV, Tadic T, Liu FF, Haibe-Kains B. Comput Biol Med. 2021 Apr 16
- Deep-CR MTLR: a Multi-Modal Approach for Cancer Survival Prediction with Competing Risks. Sejin Kim, Michal Kazmierski, Benjamin Haibe-Kains. Proceedings of Machine Learning Research, March 22, 2021.
- Automated detection of dental artifacts for large-scale radiomic analysis in radiation oncology. Arrowsmith C, Reiazi R, Welch ML, Kazmierski M, Patel T, Rezaie A, Tadic T, Bratman S, Haibe-Kains B. Phys Imaging Radiat Oncol. 2021 Apr 21.
- Biological and therapeutic implications of a unique subtype of NPM1 mutated AML. Mer AS, Heath EM, Madani Tonekaboni SA, Dogan-Artun N, Nair SK, Murison A, Garcia-Prat L, Shlush L, Hurren R, Voisin V, Bader GD, Nislow C, Rantalainen M, Lehmann S, Gower M, Guidos CJ, Lupien M, Dick JE, Minden MD, Schimmer AD, Haibe-Kains B. Nat Commun. 2021 Feb 16
- Assessment of Genetic Drift in Large Pharmacogenomic Studies. Quevedo R, Smirnov P, Tkachuk D, Ho C, El-Hachem N, Safikhani Z, Pugh TJ, Haibe-Kains B. Cell Syst. 2020 Sep 4
- Machine learning approaches to drug response prediction: challenges and recent progress. Adam G, Rampášek L, Safikhani Z, Smirnov P, Haibe-Kains B*, Goldenberg A*. NPJ Precis Oncol. 2020 Jun 15;4:19
- SYNERGxDB: an integrative pharmacogenomic portal to identify synergistic drug combinations for precision oncology. Seo H, Tkachuk D, Ho C, Mammoliti A, Rezaie A, Madani Tonekaboni SA, Haibe-Kains B. Nucleic Acids Res. 2020 May 22
- ToxicoDB: an integrated database to mine and visualize large-scale toxicogenomic datasets. Nair SK, Eeles C, Ho C, Beri G, Yoo E, Tkachuk D, Tang A, Nijrabi P, Smirnov P, Seo H, Jennen D, Haibe-Kains B. Nucleic Acids Res. 2020 May 18
- Modeling cellular response in large-scale radiogenomic databases to advance precision radiotherapy. Manem VS, Lambie M, Smith I, Smirnov P, Kofia V, Freeman M, Koritzinsky M, Abazeed ME, Haibe-Kains B, Bratman SV. Cancer Res. 2019 Sep 26
- Identifying clusters of cis-regulatory elements underpinning TAD structures and lineage-specific regulatory networks. Madani Tonekaboni SA, Mazrooei P, Kofia V, Haibe-Kains B*, Lupien M*. Genome Res. 2019 Sep 18
Graduate Students
Caryn Geady
Sejin Kim
Joseph Marsilla
Petr Smirnov
Ian Smith