New Publication from Dr. Michael Hoffman
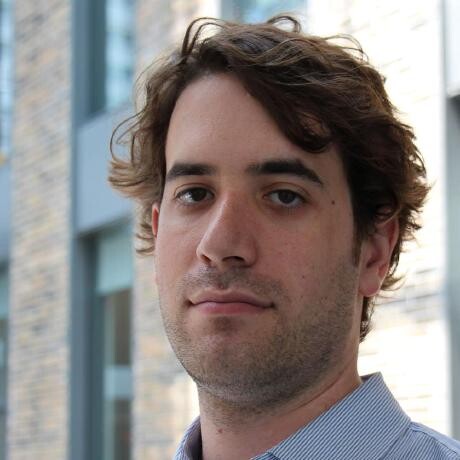
MBP researcher Dr. Michael Hoffman and several research collaborators have published a new Nature Methods article entitled 'Reproducibility standards for machine learning in the life sciences'.
Article Abstract
To make machine-learning analyses in the life sciences more computationally reproducible, we propose standards based on data, model and code publication, programming best practices and workflow automation. By meeting these standards, the community of researchers applying machine-learning methods in the life sciences can ensure that their analyses are worthy of trust.
The field of machine learning has grown tremendously within the past ten years. In the life sciences, machine-learning models are rapidly being adopted because they are well suited to cope with the scale and complexity of biological data. However, there are drawbacks to using such models. For example, machine-learning models can be harder to interpret than simpler models, and this opacity can obscure learned biases. If we are going to use such models in the life sciences, we will need to trust them. Ultimately all science requires trust1—no scientist can reproduce the results from every paper they read. The question, then, is how to ensure that machine-learning analyses in the life sciences can be trusted.
One attempt at creating trustworthy analyses with machine-learning models revolves around reporting analysis details such as hyperparameter values, model architectures and data-splitting procedures. Unfortunately, such reporting requirements are insufficient to make analyses trustworthy. Documenting implementation details without making data, models and code publicly available and usable by other scientists does little to help future scientists attempting the same analyses and less to uncover biases. Authors can only report on biases they already know about, and without the data, models and code, other scientists will be unable to discover issues post hoc.
For machine-learning models in the life sciences to become trusted, scientists must prioritize computational reproducibility2. That is to say that third parties should be able to obtain the same results as the original authors by using their published data, models and code. By doing so, researchers can ensure the accuracy of reported results and detect biases in the models.
Analyses and models that are reproducible by third parties can be examined in depth and, ultimately, become worthy of trust. To that end, we believe the life sciences community should adopt norms and standards that underlie reproducible machine learning research.