Chris McIntosh
PhD, Simon Fraser University
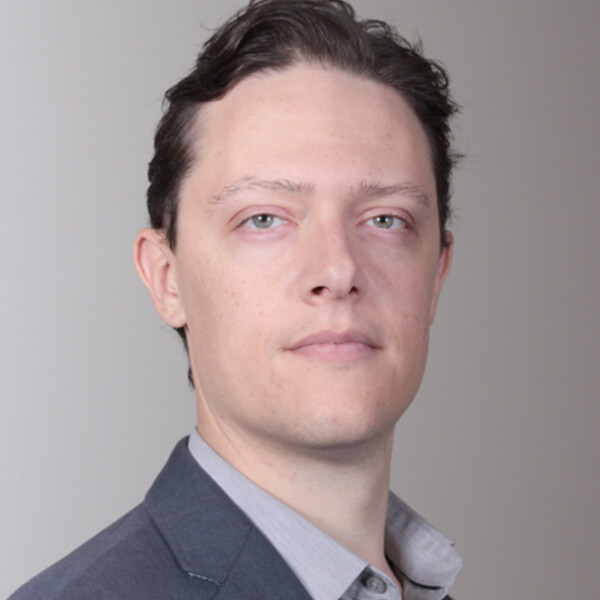
At a Glance
- The focus of my lab is on advancing the theory and application of artificial intelligence (AI) in medicine from bench-to-bedside
- Methodologies include transfer learning, meta learning, computer vision, and explainable AI to build clinically usable models
- Close collaborations with clinical stake holders yield relevant clinical problems, and large data sets with tens of thousands of images
- Developed technologies are used to prospectively provide patient care at UHN and globally
- Diseases studied include prostate cancer, lung cancer, cardiovascular disease, and associated interventions
Short Bio
Chris McIntosh is a Scientist at the Techna Institute, the Peter Munk Cardiac Centre, and the Joint Department of Medical Imaging, at the University Health Network, and an Assistant Professor in the Dept. of Medical Biophysics at the University of Toronto. Dr. McIntosh received the B.Sc. degree with honours in Computing Science from Simon Fraser University (SFU) in 2005 and his PhD in 2011 under the supervision of Dr. Ghassan Hamarneh. He is the recipient of academic awards from NSERC, CIHR, and the Michael Smith Foundation for Health Research. His dissertation received an honourable mention for the top dissertation in computer vision and medical image analysis by the Canadian Image Processing and Pattern Recognition Society. In 2010 he was a visiting researcher with the GALEN group at INRIA, Sacley, France, and Ecole Centrale de Paris. In 2012 he joined the Department of Radiation Physics as a Research Associate working in the labs of Drs. Tom Purdie and David Jaffray. In 2019 he started his own lab focusing on the theory and clinical application of AI in medicine for improving patient care including transfer learning, meta learning, computer vision, and explainable AI. Applications include deep learning for automated diagnosis, segmentation, quality assurance, and treatment planning. In particular, he and Tom Purdie’s work in radiation therapy has been commercialized and is now deployed in hospitals around the world, using AI to deliver reproducible, high quality cancer care.
Research Synopsis
Deep Learning for Automated Cancer Care: An experienced clinical team can more than double a cancer patient’s odds of survival. In particular, radiation therapy (RT) is a key part of the treatment for 40% of all cancer patients with the potential to be highly curative and effective given the right treatment plan. However, a major barrier in that effectiveness is the expertise and experience of the patient’s clinical team in his or her particular cancer. We believe that AI can help bring the expertise of the world’s best clinical teams to every patient in every hospital, saving lives and costly resources. We have developed the world’s first AI-based treatment system that is being piloted to treat prostate cancer patients at Princess Margaret Cancer Centre today, with superior results to our standard of care for over 88% of patients. Cancer treatments are generated by the AI in minutes, instead of the hours-to-days taken under the typical manual process, increasing both efficiency and quality of care. Follow-up studies are starting for additional cancer types. Through a successful licensing deal of this Canadian technology to one of the world’s largest RT software companies we are also supporting deployment across Canada and around the world.
Machine learning for Intervention Quality Assurance: The QA process in RT involves ensuring that the RT plan is safe and effective. It is a manual process where a team of medical experts reviews the plan and associated segmentations (ROIs) and examines the amount of radiation to the target and organs at risk. Our work calculates image, ROI, and plan features, and uses a novel AI models to learn expected relationships between the features for clinically effective plans. A fusion between classification and density estimation enables novel plans to be classified as erroneous due to matching pre-existing training errors or by being an atypical plan, and thus worth clinical scrutiny.
Transfer and Meta Learning: Not all diseases are amenable to traditional machine learning with only hundreds of patients due to disease rarity or the rapid evolution of their related interventions. We are investigating approaches to learn features and structures from tens of thousands of patient images to enable application to rarer diseases with smaller datasets.
Recent Publications
View recent publications of Dr. McIntosh on Google Scholar.
Graduate Students
Siham Amara Belgadi
Aly Sherif Khalifa
Cathy Ong Ly