Jüri Reimand
PhD, University of Tartu, Estonia
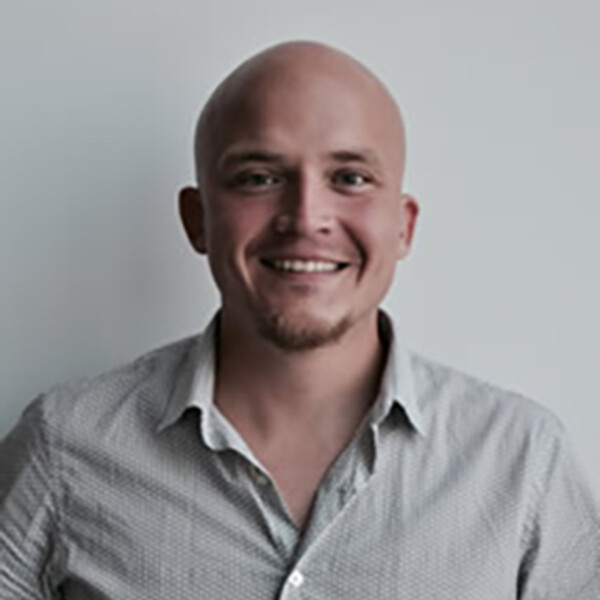
Research Synopsis
Computational network biology of cancer
Cancer is driven by somatic changes in genomes that provide cells with evolutionary advantages. Many other factors contribute to the complexity of cancer, including diversity of cancers across anatomical sites, heterogeneity within individual tumours, and genetic and environmental factors. The activities of genes, transcripts, and proteins in many common cancer types are now comprehensively profiled in massive international efforts. We need to carefully analyse these complex datasets to better understand the basic biology of cancer and its driver mechanisms, treatment opportunities, and biomarkers.
The underlying goal of our research is to interpret the molecular profiles of cancer using pathway and network information (1). Pathways and networks represent a complementary body of knowledge derived from decades of research that helps us highlight the aspects of data that are more likely representative of the underlying biology. With this assumption in mind, we develop statistical algorithms and machine-learning methods to explain -omics data, discover cancer driver genes and predictive biomarkers, interpret cancer mutations, and infer master gene regulators of cellular processes.
- Pathway enrichment analysis is a common technique used to interpret large gene lists from high-throughput experiments. We developed the g:Profiler web server (2) that detects representative biological processes and pathways in gene lists. We have often collaborated on pathway analysis, including in recent studies on brain cancer (3-5). Pathway and network information helps predict new functions to genes and characterise the biology and mechanisms active in the experiment.
- Interpreting cancer mutations is a complex task as only few mutations are cancer drivers while most are functionally inactive passengers (6). We can improve driver discovery by focusing on mutations in small sites involved in interactions of networks, as these mutations are more likely important in cancer. We used this idea to build the mutation enrichment model ActiveDriver (7) that analyses mutations in protein sites of post-translational modifications (PTMs). PTMs such as phosphorylation are involved in cellular signalling and cancer pathways. We applied ActiveDriver in the TCGA pan-cancer project to characterise the mutational landscape of signalling networks and to detect known and candidate cancer driver genes (8,9). In another study, we analysed population-wide genome variation and found that PTM sites are strongly conserved among humans and enriched in germline disease variants, emphasizing their importance in physiology and predisposition to disease (10). We recently developed the machine learning method MIMP (11) that finds mutations that disrupt or create small sequence motifs in phosphorylation sites, potentially rewiring interactions in signalling networks. These network-driven approaches help us find cancer driver mutations but also propose how they function in cancer biology.
- Gene regulatory networks of transcription factors (TFs) determine the expression of genes and thus control cellular processes and pathways. Abundant high-throughput data are available about gene expression, chromatin state, and binding sites of TFs in DNA. However accurately inferring target genes of TFs is a complex task as different types of data are often not in good agreement. Thus integrative analysis of complementary datasets helps improve reconstruction of gene regulatory networks. We have developed a data mining framework to discover gene co-expression networks from large collections of microarray datasets (12) and constructed a statistical model to predict master regulators of cellular processes from multivariate data (13,14). We are advancing these methods to decipher gene regulatory networks in hallmark processes of cancer.
Recent Publications
- Mutation Consequences and Pathway Analysis working group of the International Cancer Genome Consortium. (2015) Pathway and network analysis of cancer genomes. Nat Methods, 12, 615-621.
- Reimand, J., Kull, M., Peterson, H., Hansen, J. and Vilo, J. (2007) g:Profiler--a web-based toolset for functional profiling of gene lists from large-scale experiments. Nucleic acids research, 35, W193-200.
- Northcott, P.A., Shih, D.J., Peacock, J., Garzia, L., Morrissy, A.S., Zichner, T., Stutz, A.M., Korshunov, A., Reimand, J., Schumacher, S.E. et al. (2012) Subgroup-specific structural variation across 1,000 medulloblastoma genomes. Nature, 488, 49-56.
- Huang, X., He, Y., Dubuc, A.M., Hashizume, R., Zhang, W., Reimand, J., Yang, H., Wang, T.A., Stehbens, S.J., Younger, S. et al. (2015) EAG2 potassium channel with evolutionarily conserved function as a brain tumor target. Nat Neurosci.
- Meyer, M., Reimand, J., Lan, X., Head, R., Zhu, X., Kushida, M., Bayani, J., Pressey, J.C., Lionel, A.C., Clarke, I.D. et al. (2015) Single cell-derived clonal analysis of human glioblastoma links functional and genomic heterogeneity. Proc Natl Acad Sci U S A, 112, 851-856.
- Gonzalez-Perez, A., Mustonen, V., Reva, B., Ritchie, G.R., Creixell, P., Karchin, R., Vazquez, M., Fink, J.L., Kassahn, K.S., Pearson, J.V. et al. (2013) Computational approaches to identify functional genetic variants in cancer genomes. Nat Methods, 10, 723-729.
- Reimand, J. and Bader, G.D. (2013) Systematic analysis of somatic mutations in phosphorylation signaling predicts novel cancer drivers. Molecular systems biology, 9, 637.
- Tamborero, D., Gonzalez-Perez, A., Perez-Llamas, C., Deu-Pons, J., Kandoth, C., Reimand, J., Lawrence, M.S., Getz, G., Bader, G.D., Ding, L. et al. (2013) Comprehensive identification of mutational cancer driver genes across 12 tumor types. Sci Rep, 3, 2650.
- Reimand, J., Wagih, O. and Bader, G.D. (2013) The mutational landscape of phosphorylation signaling in cancer. Sci Rep, 3, 2651.
- Reimand, J., Wagih, O. and Bader, G.D. (2015) Evolutionary constraint and disease associations of post-translational modification sites in human genomes. PLoS Genet, 11, e1004919.
- Wagih, O., Reimand, J. and Bader, G.D. (2015) MIMP: predicting the impact of mutations on kinase-substrate phosphorylation. Nat Methods, 12, 531-533.
- Adler, P., Kolde, R., Kull, M., Tkachenko, A., Peterson, H., Reimand, J. and Vilo, J. (2009) Mining for coexpression across hundreds of datasets using novel rank aggregation and visualization methods. Genome biology, 10, R139.
- Reimand, J., Vaquerizas, J.M., Todd, A.E., Vilo, J. and Luscombe, N.M. (2010) Comprehensive reanalysis of transcription factor knockout expression data in Saccharomyces cerevisiae reveals many new targets. Nucleic acids research, 38, 4768-4777.
- Reimand, J., Aun, A., Vilo, J., Vaquerizas, J.M., Sedman, J. and Luscombe, N.M. (2012) m:Explorer: multinomial regression models reveal positive and negative regulators of longevity in yeast quiescence. Genome biology, 13, R55.
Graduate Students
Masroor Bayati
Chi Lok Kevin Cheng