Anne Martel
PhD, Sheffield, UK
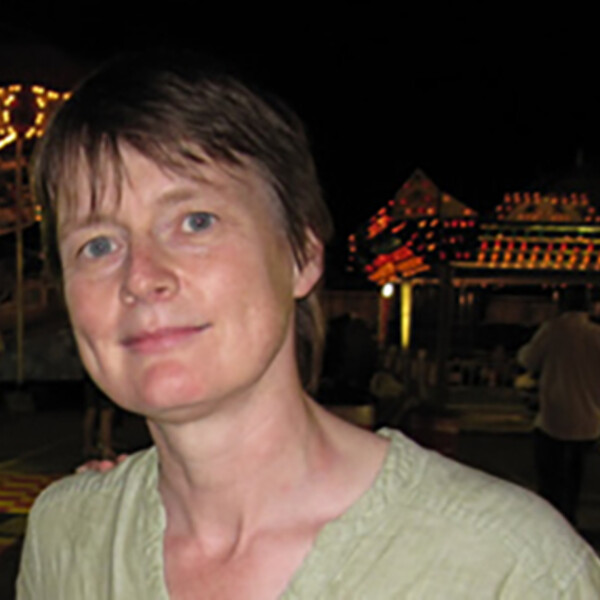
At A Glance
- The lab focus is on the development of machine learning and AI techniques applied to radiological and digital pathology images for detection, diagnosis and prediction
- We are particularly interested in applying weakly supervised and self supervised methods to overcome the problems associated with acquiring large cohorts of labelled data
- Our work concentrates on oncology applications, particularly breast cancer
Short Bio
Dr Martel is a Senior Scientist in the Physical Sciences platform at Sunnybrook Research Institute and the Tory Family Chair in Oncology. She is also a Vector Faculty Affiliate. Her research program is focused on medical image and digital pathology analysis, particularly on applications of machine learning for segmentation, diagnosis, and prediction/prognosis. In 2006 she co-founded Pathcore, a software company developing complete workflow solutions for digital pathology.
Dr Martel is an active member of the medical image analysis community and is a fellow of the MICCAI Society which represents engineers and computer scientists working in this field. She has served as board member of MICCAI and is currently on the editorial board of the journal Medical Image Analysis.
Research Synopsis
Self-supervised methods in medical imaging: It is expensive and time consuming to generate sufficient labelled data to train a machine learning model. We are exploring the use of self-supervised and contrastive learning approaches to pretrain models using large unlabelled datasets. We were the first group to demonstrate that resnet models pretrained on pathology images using SimCLR are able to learn downstream computational pathology tasks more accurately and with a fraction of the labels required by models pretrained using imageNet and the resnet model we released publicly has been widely downloaded and used.
Survival prediction using radiomics and deep learning: When deciding what treatment to offer cancer patients, there is usually a trade-off between aggressive therapy which has a higher likelihood of prolonging life at the cost of increased side effects from the treatment itself, versus less intensive therapy that may lead to a shorter survival time but better quality of life for the patient. Radiomics and AI can be used to predict outcomes based on the appearance of the tumor on medical imaging but datasets are small and there is a real risk of over fitting models. We are developing methods of identifying imaging phenotypes using unlabelled data in order to address this problem.
AI applied to breast cancer: We have several CIHR funded studies underway to train AI models for risk prediction from MRI images, predict the presence of occult invasive cancer from both digital pathology and mammography images and to predict recurrence free survival from digital pathology images and other clinical data.
Recent Publications
- Chen, J., Cheung, H. M. C., Karanicolas, P. J., Coburn, N. G., Martel, G., Lee, A., Patel, C., Milot, L., & Martel, A. L. (2023). A radiomic biomarker for prognosis of resected colorectal cancer liver metastases generalizes across MRI contrast agents. Frontiers in Oncology, 13, 898854. https://doi.org/10.3389/fonc.2023.898854
- Srinidhi, C. L., Kim, S. W., Chen, F.-D., & Martel, A. L. (2022). Self-supervised driven consistency training for annotation efficient histopathology image analysis. Medical Image Analysis, 75, 102256. https://doi.org/10.1016/j.media.2021.102256
- Ciga, O., Xu, T., & Martel, A. L. (2022). Self supervised contrastive learning for digital histopathology. Machine Learning with Applications, 7, 100198. https://doi.org/10.1016/j.mlwa.2021.100198
- Kuling, G., Curpen, B., & Martel, A. L. (2022). BI-RADS BERT and Using Section Segmentation to Understand Radiology Reports. Journal of Imaging, 8(5), 131. https://doi.org/10.3390/jimaging8050131
- Ciga, O., Xu, T., Nofech-Mozes, S., Noy, S., Lu, F., & Martel, A. L. (2021). Overcoming the limitations of patch-based learning to detect cancer in whole slide images. Scientific Reports, 11(1), 8894. https://doi.org/10.1038/s41598-021-88494-z
- Petrick, N., Akbar, S., Cha, K. H., Nofech-Mozes, S., Sahiner, B., Gavrielides, M. A., Kalpathy-Cramer, J., Drukker, K., Martel, A. L., & BreastPathQ Challenge Group, for the. (2021). SPIE-AAPM-NCI BreastPathQ challenge: an image analysis challenge for quantitative tumor cellularity assessment in breast cancer histology images following neoadjuvant treatment. Journal of Medical Imaging, 8(03), 034501. https://doi.org/10.1117/1.JMI.8.3.034501
Visit the Google Scholar profile of Dr. Anne Martel.
Graduate Students
Jianan Chen
Geoff Klein
Grey Kuling
Matthew McNeill
Vishwesh Ramanathan
Phoenix Wilkie
Tony Xu