Igor Jurisica
PhD, University of Toronto
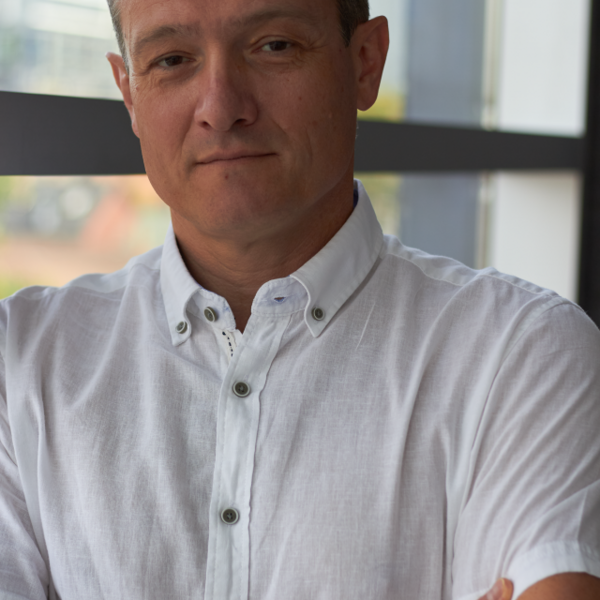
Specific Research Interests
- Integrative computational biology
- Data mining
- Machine learning
- AI and explainable models in chronic diseases.
Short Bio
Igor Jurisica, PhD, DrSc is a Senior Scientist at Osteoarthritis Research Program, Division of Orthopedic Surgery, Schroeder Arthritis Institute and Data Science Discovery Centre for Chronic Diseases, Krembil Research Institute, Professor at University of Toronto and Visiting Scientist at IBM CAS. Since 2015 he has served as Chief Scientist at the Creative Destruction Lab, Rotman School of Management, and since 2021 he is a scientific director of the World Community Grid.
His research focuses on integrative informatics and the representation, analysis and visualization of high-dimensional data to identify prognostic/predictive signatures, determine clinically relevant combination therapies, and develop accurate models of drug mechanism of action and disease-altered signaling cascades. He has published extensively on data mining, visualization and integrative computational biology, including multiple papers in Science, Nature, Nature Medicine, Nature Methods, J Clinical Oncology, J Clinical Investigations.
He has been included in Thomson Reuters 2014, 2015 & 2016 lists of Highly Cited Researchers (http://highlycited.com), and The World's Most Influential Scientific Minds: 2015 & 2014 Reports. In 2019, he has been recognized as the Top 100 AI Leaders in Drug Discovery and Advanced Healthcare list (Deep Knowledge Analytics, http://analytics.dkv.global). In 2023, he has been included in the Top 100 AI in Oncology leaders list (Deep Knowledge Analytics, https://platform.dkv.global/map/reports/ai-in-oncology-leaders).
Research Synopsis
The primary research focus is on integrative computational biology, and representation, analysis and visualization of high dimensional data generated by high-throughput biology experiments. Of particular interest is the use of comparative analysis in the mining of different dataset types such as protein-protein interaction, gene/protein expression profiling, microRNA:target, drug:target, to identify prognostic/predictive signatures, determine clinically relevant combination therapies, and develop accurate models of drug mechanism of action and disease-altered signaling cascades. Importantly, understanding these mechanisms would not only help to treat the disease more accurately, but to provide personalized options for disease prevention.
My team has built and continues to expand powerful computational infrastructure, unique algorithms and data integration portals for physical protein interactions, pathways, ncRNAs, transcription factors, prognostic signatures, drug predictions, and bio-assay data analysis. Our feature-rich NAViGaTOR and graph algorithms complement these portals set to enable effective visual data mining and generation of explainable disease models. We continue to make these databases and algorithms publicly available (http://www.cs.utoronto.ca/~juris/tools.html).
In September 2021 we have taken control of the IBM World Community Grid, (WCG; https://www.worldcommunitgrid.org). WCG is an open science, high performance computing platform enabling advanced open science and open data research that benefits humanity. It is a global, heterogeneous, distributed cloud infrastructure that runs on the secure BOINC platform developed at the University of Berkeley (https://boinc.berkeley.edu). WCG is one of the world’s largest citizen science platforms, thanks to over 810,400 volunteers globally who have donated over 7,651,164 devices contributing more than 2.5 million CPU-years of computational power. This enabled WCG to support 31 computationally intensive research projects to date, including investigation into improved treatments for cancer, HIV/AIDS, COVID19, and under-researched tropical dis-eases such as ZIKA, as well as exploration of sustainable energy solutions (https://www.worldcommunitygrid.org/research/projects.s?proj=comp).
For further information, please visit our website.
Recent Publications
- Wong SWH, Pastrello C, Kotlyar M, Faloutsos C, Jurisica I. USNAP: Fast unique dense region detection and its application to lung cancer, Bioinformatics, 39(8):btad477, 2023
- Cente M, Perackova J, Peracek P, Majdan M, Toth I, Mikulic M, Hanes J, Porubska S, Spajdel M, Kazickova B, Jurisica I, Filipcik P. Association of Nonconcussive Repetitive Head Impacts and Intense Physical Activity With Levels of Phosphorylated Tau181 and Total Tau in Plasma of Young Elite Soccer Players. JAMA Netw Open. 6(3):e236101, 2023.
- Houschild AC, Pastrello C, Ekaputeri GKA, Bethune-Waddell D, Abovsky M, Ahmed Z, Kotlyar M Lu R, Jurisica I. MirDIP 5.2: tissue context annotation and novel microRNA curation, Nuncl Acids Res, 51(D1):D217-D225, 2023.
- G. Agapito, Y. Niu, C. Pastrello, I. Jurisica. Pathway integration and annotation: building a puzzle with non matching pieces and no picture to follow, Briefings in Bioinformatics, 23(5):bbac368, 2022.
- Cente M, Matyasova K, Csicsatkova N, Tomikova A, Porubska S, Niu Y, Majdan M, Filipcik P, Jurisica I. Traumatic MicroRNAs: Deconvolving the Signal After Severe Traumatic Brain Injury. Cell Mol Neurobiol. 2022 Jul 19. doi: 10.1007/s10571-022-01254-z. Epub ahead of print
- Pastrello C, Abovsky M, Lu R, Ahmed Z, Kotlyar M, Veillette C, Jurisica I, Osteoarthritis Data Integration Portal (OsteoDIP): A web-based gene and non-coding RNA expression database, Osteoarthritis and Cartilage Open, 4(1): 100237, 2022.
- Sandmo SB, Matyasova K, Filipcik P, Cente M, Koerte IK, Pasternak O, Andersen TE, Straume-Næsheim TM, Bahr R, Jurisica I. Changes in circulating microRNAs following head impacts in soccer. Brain Inj, 36(4):560-571, 2022
- Tokar T, Pastrello C, Abovsky M, Rahmati S, Jurisica I. miRAnno—network-based functional microRNA annotation, Bioinformatics, 38(2):592–593, 2022.
- Kotlyar M, Pastrello C, Ahmed Z, Chee J, Varyova Z, Jurisica I, IID 2021: Towards context-specific protein interaction analyses by increased coverage, enhanced annotation and enrichment analysis. Nucl Acids Res, 50(D1):D640-D647, 2022.